Understanding the Role of Statistics in Cricket Betting
Statistics play a crucial role in cricket betting, offering insights beyond gut feelings and guesses.
Importance of Statistical Analysis
Statistical analysis helps bettors make informed decisions in cricket betting. It enables analysis of player performance, team dynamics, and historic outcomes, offering a data-driven foundation for predictions.
Key Reasons
- Player Performance Analysis: Statistics reveal batting averages, bowling economies, and other critical performance indicators.
- Historic Match Data: Past match outcomes can highlight patterns and tendencies, aiding in more accurate predictions.
- Risk Management: Analyzing past data helps in assessing potential risks, improving decision-making during wagers.
Common Statistical Metrics Used in Betting
Several key metrics assist in cricket betting. Understanding these can refine betting strategies.
- Batting Average: Measures runs scored per dismissal. Example: Player A’s average is 45, indicating consistency.
- Strike Rate: Represents runs scored per 100 balls faced. Example: A strike rate of 130 means 130 runs per 100 balls.
- Bowling Average: Calculates runs conceded per wicket taken. Example: A bowler with an average of 20 is efficient.
- Economy Rate: Indicates runs conceded per over. Example: An economy rate of 6 means 6 runs per over bowled.
- Head-to-Head Records: Past encounters between teams offer insights into potential match outcomes.
These metrics assist bettors in crafting well-informed and strategic wagers based on quantitative evidence.
How Bookmakers Use Statistics
Bookmakers rely heavily on statistics to set and adjust betting odds. They use detailed data analysis to ensure odds reflect the most likely outcomes and maximize their profits.
Setting the Odds
Bookmakers base their odds on historical performance data. They analyze players’ batting and bowling averages, strike rates, economy rates, and head-to-head records.
For instance, a batsman with a high average and strike rate against a particular team likely gets favorable odds for top scorer.
Similarly, bowlers with a low economy rate and high wicket count against opponents have shorter odds for most wickets. This statistical modeling helps create accurate and attractive betting markets.
Adjusting the Bet Lines
Bookmakers constantly adjust bet lines to balance their books. They monitor real-time match data, player injuries, and weather conditions.
If key players get injured or if the pitch conditions change drastically, odds get recalibrated to reflect these new variables. For example, if a star bowler pulls out just before the match, the odds may shift in favor of the batting team.
This dynamic adjustment ensures bookmakers manage risk and maintain profitability.
Statistical Models Popular in Cricket Betting
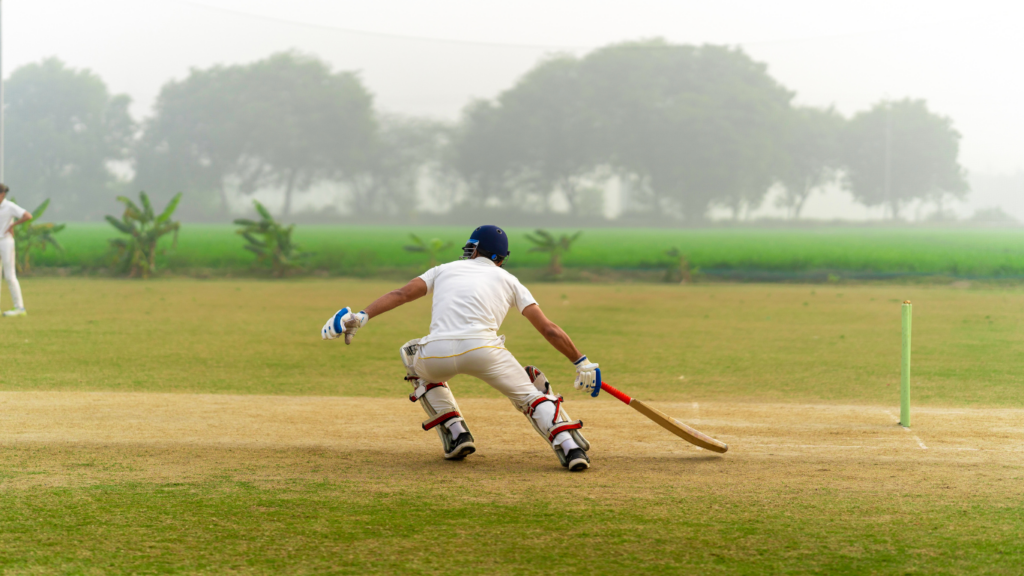
Statistics play a crucial role in cricket betting. Popular statistical models help bookmakers and bettors make accurate predictions and decisions.
Predictive Modeling Techniques
Predictive modeling techniques use historical data to forecast future outcomes. Regression analysis predicts player performance, examining variables like:
- past scores,
- pitch conditions,
- opposition strength.
For example, linear regression analyzes player runs in different conditions to estimate scores in upcoming matches.
Machine learning models, like Random Forests and Neural Networks, handle massive data volumes, identifying complex patterns and improving prediction accuracy.
Decision trees segment data into smaller subsets for easy ‘yes’ or ‘no’ decision-making. Bayesian models update probabilities as more information emerges, refining predictions on player form or match outcomes.
Predictive models leverage these techniques to enhance betting strategies in cricket, making data-driven predictions more reliable.
Real-Time Data Analysis
Real-time data analysis adapts quickly to evolving match conditions. In-play betting uses real-time metrics like wicket falls, run rates, and player fitness to adjust odds dynamically.
For instance, if a key player gets injured, data analytics tools update odds immediately. Streaming data technologies like Apache Kafka process ongoing match data for real-time insights.
These insights allow bettors to react quickly to on-field changes, enhancing their betting strategy. Conditional probability calculations assess event likelihoods, such as the probability of winning after losing early wickets.
Real-time analysis turns raw match data into actionable insights, significantly impacting cricket betting decisions by providing up-to-the-minute information.
Impact of Statistics on Betting Success
Statistics significantly enhance success in cricket betting. Bettors use data-driven approaches to gain an edge over others.
Case Studies and Success Stories
Successful bettors leverage statistics effectively. An example is the 2019 Cricket World Cup, where data analysts accurately predicted England’s win based on player performance statistics and weather conditions.
Bettors who followed these insights achieved higher returns.
Another case involves the Indian Premier League (IPL). Analysts used machine learning models to forecast match outcomes. Bettors who incorporated these predictions saw improved accuracy in their bets, resulting in substantial profits.
In a study by the Journal of Sports Analytics, bettors using statistical models increased their win rates by 15% compared to those relying on intuition.
These instances highlight how data-driven strategies contribute to better betting outcomes.
Limitations and Challenges
Despite its advantages, using statistics in cricket betting has limitations. Data accuracy is a major challenge. If historical data is incomplete or biased, predictions can be flawed.
Variables like weather, pitch conditions, and player injuries add complexity.
Even the best models can’t account for every unforeseen factor. Bettors need to understand these limitations to avoid over-relying on statistical predictions.
Adaptability is key, as the dynamic nature of live matches demands real-time data analysis. Bettors must adjust strategies based on live metrics, which isn’t always feasible under time constraints.
While statistics play a crucial role, bettors should combine data insights with situational awareness and adaptability to maximize success in cricket betting.
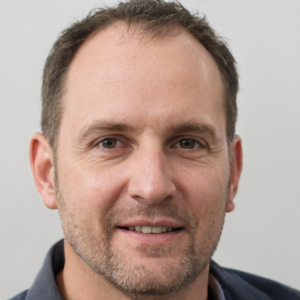
Charlessen Hitchcockiee
Charlessen Hitchcockiee is the visionary founder behind Play Daily Win Big, a leading platform dedicated to delivering the latest in betting and gaming news. With a deep-rooted passion for the gaming industry, Charlessen has built a reputation for providing insightful analysis and valuable updates that cater to both casual players and industry experts. His extensive knowledge spans market trends, regulatory changes, and strategic gaming tips, making Play Daily Win Big a trusted resource for its audience.
Based in San Francisco, Charlessen is known for his analytical approach and keen ability to predict market shifts. His commitment to quality content and timely updates reflects his dedication to the gaming community. Through Play Daily Win Big, he aims to enhance the gaming experience by offering accurate, relevant information that helps enthusiasts and professionals stay ahead in the dynamic world of betting and gaming.